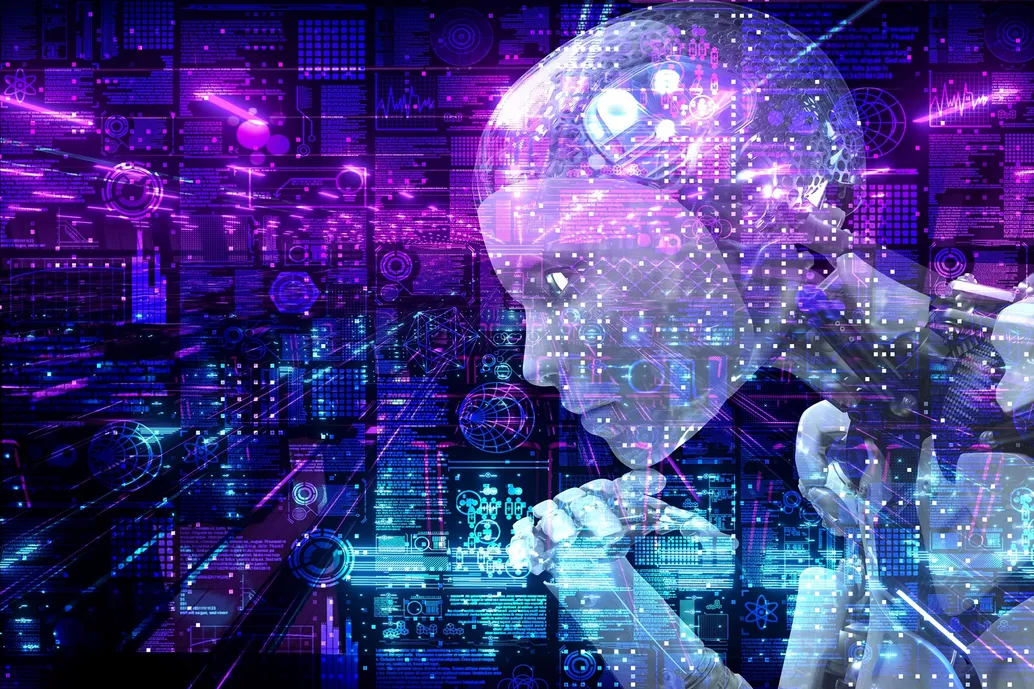
Des chercheurs de l’Université Duke ont découvert que les algorithmes d’apprentissage automatique peuvent acquérir de nouveaux degrés de transparence et de compréhension des propriétés des matériaux après leur avoir enseigné des notions de physique.
L’incorporation de la physique établie dans les algorithmes de réseaux neuronaux les aide à découvrir de nouvelles idées sur les propriétés des matériaux.
Selon des chercheurs de l’Université Duke, l’incorporation de la physique connue dans les algorithmes d’apprentissage automatique peut aider les énigmatiques boîtes noires à atteindre de nouveaux niveaux de transparence et de compréhension des caractéristiques des matériaux.
Les chercheurs ont utilisé un algorithme sophistiqué d’apprentissage automatique dans l’un des premiers efforts de ce type pour identifier les caractéristiques d’une classe de matériaux d’ingénierie connus sous le nom de métamatériaux et pour prédire comment ils interagissent avec les champs électromagnétiques.
L’algorithme a été essentiellement forcé de montrer son travail puisqu’il devait d’abord prendre en compte les restrictions physiques connues du métamatériau. La méthode a non seulement permis à l’algorithme de prédire les propriétés du métamatériau avec une grande accuracy, but it also did it more quickly and with additional insights than earlier approaches.
Silicon metamaterials such as this, featuring rows of cylinders extending into the distance, can manipulate light depending on the features of the cylinders. Research has now shown that incorporating known physics into a machine learning algorithm can reveal new insights into how to design them. Credit: Omar Khatib
The results were published in the journal Advanced Optical Materials on May 13th, 2022.
“By incorporating known physics directly into the machine learning, the algorithm can find solutions with less training data and in less time,” said Willie Padilla, professor of electrical and computer engineering at Duke. “While this study was mainly a demonstration showing that the approach could recreate known solutions, it also revealed some insights into the inner workings of non-metallic metamaterials that nobody knew before.”
Metamaterials are synthetic materials composed of many individual engineered features, which together produce properties not found in nature through their structure rather than their chemistry. In this case, the metamaterial consists of a large grid of silicon cylinders that resemble a Lego baseplate.
Depending on the size and spacing of the cylinders, the metamaterial interacts with electromagnetic waves in various ways, such as absorbing, emitting, or deflecting specific wavelengths. In the new paper, the researchers sought to build a type of machine learning model called a neural network to discover how a range of heights and widths of a single-cylinder affects these interactions. But they also wanted its answers to make sense.
“Neural networks try to find patterns in the data, but sometimes the patterns they find don’t obey the laws of physics, making the model it creates unreliable,” said Jordan Malof, assistant research professor of electrical and computer engineering at Duke. “By forcing the neural network to obey the laws of physics, we prevented it from finding relationships that may fit the data but aren’t actually true.”
The physics that the research team imposed upon the neural network is called a Lorentz model — a set of equations that describe how the intrinsic properties of a material resonate with an electromagnetic field. Rather than jumping straight to predicting a cylinder’s response, the model had to learn to predict the Lorentz parameters that it then used to calculate the cylinder’s response.
Incorporating that extra step, however, is much easier said than done.
“When you make a neural network more interpretable, which is in some sense what we’ve done here, it can be more challenging to fine-tune,” said Omar Khatib, a postdoctoral researcher working in Padilla’s laboratory. “We definitely had a difficult time optimizing the training to learn the patterns.”
Once the model was working, however, it proved to be more efficient than previous neural networks the group had created for the same tasks. In particular, the group found this approach can dramatically reduce the number of parameters needed for the model to determine the metamaterial properties.
They also found that this physics-based approach to artificial intelligence is capable of making discoveries all on its own.
As an electromagnetic wave travels through an object, it doesn’t necessarily interact with it in exactly the same way at the beginning of its journey as it does at its end. This phenomenon is known as spatial dispersion. Because the researchers had to tweak the spatial dispersion parameters to get the model to work accurately, they discovered insights into the physics of the process that they hadn’t previously known.
“Now that we’ve demonstrated that this can be done, we want to apply this approach to systems where the physics is unknown,” Padilla said.
“Lots of people are using neural networks to predict material properties, but getting enough training data from simulations is a giant pain,” Malof added. “This work also shows a path toward creating models that don’t need as much data, which is useful across the board.”
Reference: “Learning the Physics of All-Dielectric Metamaterials with Deep Lorentz Neural Networks” by Omar Khatib, Simiao Ren, Jordan Malof and Willie J. Padilla, 13 May 2022, Advanced Optical Materials.
DOI: 10.1002/adom.202200097
This research was supported by the Department of Energy (DESC0014372).